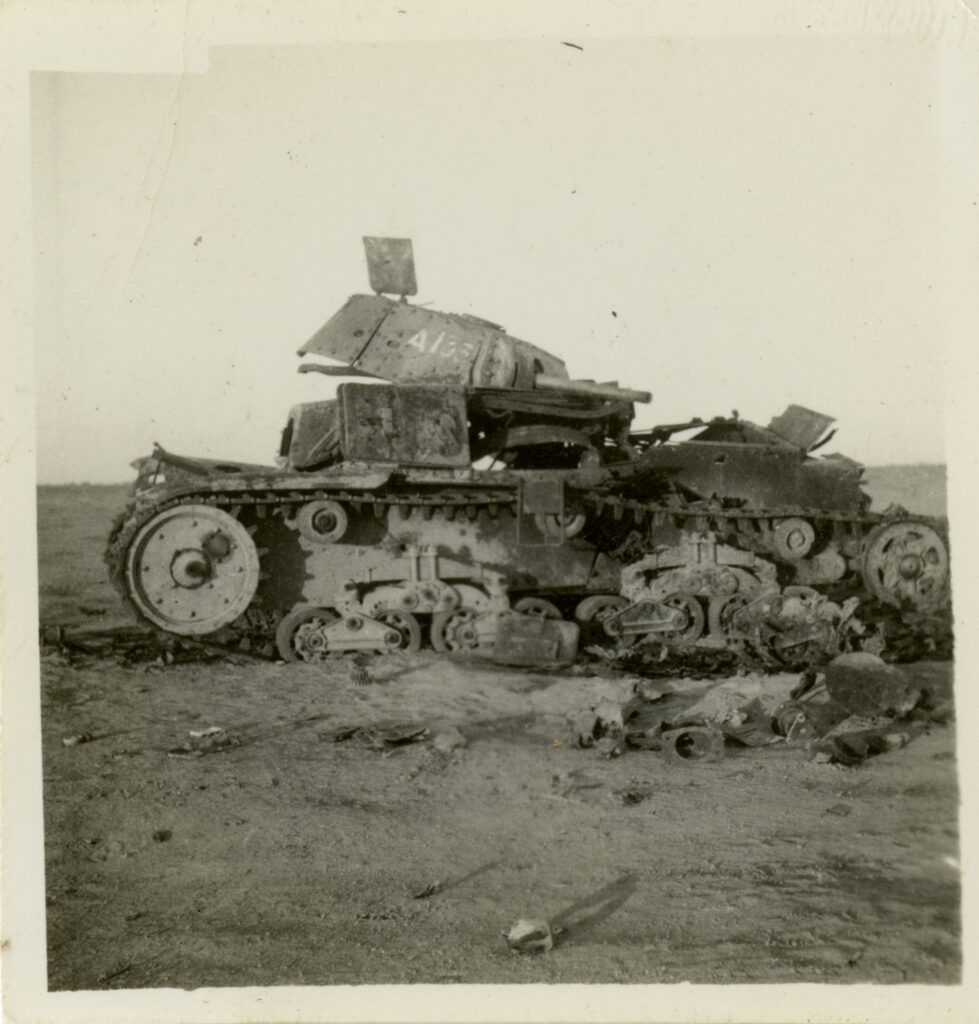
You’ve just reached into a large opaque jar and pulled out the number 87. I could add some other structure, that the biggest number is 100, or that odd numbers are twice as common as even, and then ask clearly answerable questions. But what if we know nothing more than what we’ve seen: a ball from the jar with the number 87? What’s the biggest number in the jar? Are there even other numbers in the jar? Or you’ve reached in and pulled out a purple marble, how many colors of marble are there? Are they all round? Are there any numbers in the jar? Some of these have actual answers, and that’s important because they link to a bigger question:
What do our philosophies of statistics fill in about our world when they’ve seen almost nothing from it?
Or put another way: just how much can you know about the world with nearly no data and nearly no theory? I’m in social science, so it’s a real thrill when I find knowledge I can trust. And to do it from nearly nothing is astonishing. You can get the shape of the world from just 1 or even 0 observations. As an empirics-first person, I’m surprised to hear myself say it, but there’s so much you already know about the world when you don’t know nearly nothing. I’ve been collecting examples for year. And these examples have actually helped me do normal things, like find my keys, charge my phone, and, thanks to German tanks, take a long, hot shower.
The foggy sea, the German tank problem, and showers at a campsite.
You’ve been wandering for weeks, no map, through a foggy landscape. You reached a body of water, knowing nothing about how large it is, and decided to cross it with your inflatable raft. It’s been about 10 minutes already, so you know that this body of water is bigger than a creek, but it could be a lake, sea, or ocean. It could be another 2 minutes to the other side, or two months. Knowing nothing, what’s your best guess for the total size of the lake?
This is a continuous version of a famous statistical problem called the German tank problem. The Allies need to estimate German tank production. As they captured and examined German tank hulls, they found that their engines had serial numbers. So if you’ve captured a tank with the number 200 on the engine, you immediately know that there are at least 200 tanks. But does that also tell you anything about the total number of tanks? It does, and that’s fascinating: it’s a hint of everything you know when you know almost nothing.
And it’s not just a matter of theory. I was at a camping ground with spotty hot water. Some days there was as much as you want, and others it only lasted long enough to get your hopes up, just one minute or two, before getting icy. So if you’ve been in the shower for one minute, you know that there was at least one minute of hot water available, but do you now know anything about how much hot water is left?
Amazingly, you do. And that’s useful to know. If you need 10 minutes to wash your hair, you’ll be in a bind if the water goes icy in five. And absent the magic answer, it’s not entirely clear what you should do. Play it safe and never wash your hair? Roll the dice and have soap in your eyes when the water turns cold? Well there’s a solution to this problem, the German Tank Problem, and to the problem of the foggy sea: Your best guess at any moment is that you’re halfway through. So if you’ve been rowing for 10 minutes, then your best guess in this moment is that there are ten minutes of rowing. And every minute after that you best guess will go up (not down!). If you’ve captured tank #350 then your best guess in this moment is that there are another 350 tanks, for a total of 700. And if you don’t know if you’ll have five minutes of hot water in the shower, spend the first five minutes showering cautiously, as if the hot water could end at any moment. At the five minute mark you have a legitimate reason to feel confident that you’ll have five more minutes to wash your hair.
That’s what I did, and it worked!
Connection to science
The scientific method in the West comes out of several centuries of debate: can you know the world by reason alone? By observation alone? To skip past a lot of arguing, the answer is “both” via the scientific method, a procedure for bringing reason and observation into dialogue. But in practice they’re always in balance, and whether to center theory or data differs so much by discipline. In some areas of knowledge, like physics, data drapes beautifully off the framework of theory. In others, with phenomena that are too complex for elegant theories (e.g. social science), theory does a much more sorry job at propping up the data, so you end up using the data as its own model. that explains the role of machine theory, information theory, and statistics. As a social scientist your theories aren’t nearly good enough to really predict outcomes, and you spend a lot of time with statistics, the part of math that turns data into knowledge.
The conventional statistics that social scientists are taught can be called “small-n” statistics because it was developed in the early 20th century when it was costly to collect many independent observations (n represent your total number of observations). You had to squeeze every bit of insight from the couple dozen n you could get. The computing of the 21st century brought us big data and a switch to an alterantive philosophy of statistics that could leverage large n.
Within that frame, this exercise, “what you know when you know nothing” is a bit of a throwback. We drop from large-n statistics, down past small-n statistics, to the very smallest-n statistics of n=1.
Relevance to the philosophy of statistics
There isn’t just one statistics of n=1, and there’s actually a different answer to the German Tank problem. There are two alternative philosophies of statistics: the frequentist and Bayesian paradigms. There is a clear formal difference that is hard to cast intuitively, but narratively frequentism develops statistics by estimating what will happen from what has happened, while Bayesianism understands statistics as a problem of estimating what will happen from an observer’s beliefs about what has happened. Instead of trying to figure out how many tanks are in this world, the Bayesian observer imagines a range of possible worlds, in which the Germans have built everything from 2 to 20,000 tanks, and they work to determine which of those worlds we’re in. It may sound like a subtle distinction, but philosophically it’s big and mathematically it’s big. And, as we’ll see, the different philosophies predict very different numbers of tanks.
Between the two, Bayesianism is ascendant today because it wasn’t feasible to use before the ubiquity of cheap computing, but one isn’t better than the other. They are both ways of writing models, and all models are wrong.
One practical difference: the Bayesian approach is better for handling the complexity that comes with a lot of data, while the frequentist approach was developed for the era of “small-n” statistics, when the challenge was usually to learn as much as you could from very little data. And because the German Tank Problem is a “very very small data” problem, the frequentist answer is better. The frequentist answer is the one I’ve described, that what you’ve observed is half of the total. The Bayesian solution is that what you’ve observed is the total: if the largest serial number you’ve seen is #350, then your best guess is that there are only 350 tanks, because, assuming tanks are costly to produce, a world of 350 tanks is the one with the most evidence.
But the Bayesian way of thinking has it’s own place as well in revealing what we know when we know nothing.
How to find your keys
I lost my keys on the ride to the gym, somewhere on a mile-long stretch, and it was dark by the time I came out of the gym and realized it. I didn’t want to wait till morning, and I didn’t want to backtrack slowly and spend a half hour looking carefully. After all, they could have been anywhere. So I thought about it. Are my keys equally likely to be anywhere along the mile stretch? Or are they more likely to be in some places than others? I could be in a world in which it was very unlikely that I would lose my keys at all. In that world they really could be anywhere. I could also be in a world in which they were just waiting to be lost, as if they were scotch-taped to the outside of my side pocket. In that world I probably lost them right away, walking down my steps. I didn’t know which world I was in, or which of all the worlds in between, but in more of those worlds my keys were near my front door. So instead of searching slowly back home from the gym, I decided to ride straight home and start the search at my front door. My keys turned out to be right there. In most possible worlds, you lost your keys as soon as they were loseable, and they are most likely to be wherever you last remember having them or moving them. The power of Bayesian reasoning is that you can reason to that, and you can prove it too, which some friends helped me do in another post.
How much charge is on your phone?
If you look at your phone, it’s unlikely that it’s at 68% charge. But unless it’s constantly dead (0%), or constantly charging (100%), it is more likely to be at 68% than anything else. If you charge intermittently, but enough to stay above empty, and not enough to keep at full, then you can think of it this way: It’s morning and you’re at 100%. By evening it would be at 0%, but you charge in little moments during the day. We’ll say that you took a step down every time you were drained by a point, and a step up every time you charged by a point. We’ll say that when you hit 100% you always unplug (you can’t take a step up over 100).
A question is: how many ways are there to take 100 steps from 100%; how many paths are there in all the different combinations of up and down steps? And for any given charge level, how many ways are there to that point from 100% that involve exactly 100 steps? 0% only has one path leading to it: there is only one way to take 100 steps down from 100%. The 2% level has about 100 paths leading to it: a hundred ways to take 98 steps down with a little goosestep up and down at some point between the top and bottom. There are a lot of paths from 100% back to 100%: you can go down 50 and up 50, you can go down and up 50 times, you can go down and up by 4 then 7 then 15.
With these ideas, we’re now to our key question. Which charge level between 0 and 100 has the greatest number of paths leading to it? The 68% charge level is the one with the greatest number of paths leading to it (0% has the fewest). Another way of saying the same thing: if you randomly generate paths of length 100, up and down, over and over, the number you’ll land on the most is 68%. Not by a lot, but if I know nearly nothing about your phone—it’s got about a day of charge, it’s near the end of the day, you’re on the move enough that it’s often but not usually plugged in—the least bad guess is that you’re at about 60-70% charge by the end of the day.
The orthography of number
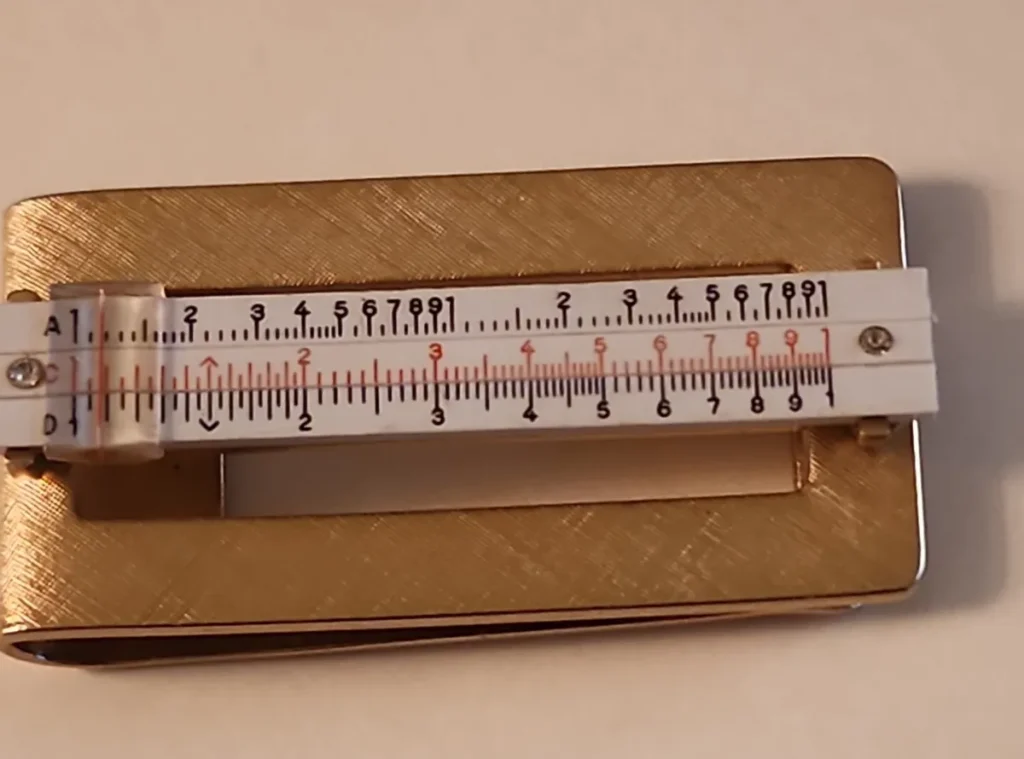
In the next book you pick up, keep an eye out for the first number you see, not spelled out but in digits. Will it be big, or little? Even or odd? What can we say about the numbers that are dealt to us, numbers about anything: dollars, marbles, people, fish? The fun thing about pure reason is a) you will learn something interesting, and b) you won’t get to choose what. According to Benford’s Law, the next number you see, big or small, is most likely to start with a 1. A number starting with 1 is 30%! That ends up being about 12% more likely than 2, which is about 5% more likely than 3, and so on down to 9, which initiates only 4.6% of numbers, not 11% like you’d expect (11=100/9 digits; you don’t divide by 10 because in Arabic numerals the only number than can start with the tenth digit, 0, is 0). I don’t understand it perfectly but it’s got something to do with there being more small numbers than big numbers, with logarithms, and with Arabic numerals. They come together to give 1’s center-stage. I don’t know if this is a metaphor or the actual explanation, but if you look at the way a slide rule gives physical space to each digit according to the logarithmic way of representing “bigness”, you’ll see that 1 gets more space than any of the others, and in that way gets more real estate in our lives, with pride of place on the far left of most written numbers.
It might sound far fetched to say, but pure reason, charged with statistical theory, and seeded with one observation, can help you shower comfortably, find your keys quickly, and keep your phone alive. It can probably also help you brush your teeth, clean your windows, and wash the dishes; let me know what you find.